Contact Event Detection for Robotic Oil Drilling
Stanford University
Fall 2012 - Fall 2013
I worked in collaboration with Alice Wu and Robotic Drilling Systems, AS (RDS) on this project, which focused primarily on the detection of unintentional slips between robotic end effectors (grippers and roughnecks) and their payloads: large, heavy metal pipes.
Robotic drilling has become an increasingly desirable goal as the oil industry seeks to enhance operator safety and reduce costs. RDS developed an autonomous robot for unmanned exploration drilling in harsh environments and sought our help developing a contact event detection method. Contact and slip event detection are crucial for reliable robotic manipulation as they allow recognition of successfully executed tasks and enable quick and appropriate reactions to unanticipated ones, such as a pipe slipping in a gripper.
We developed a scheme that identifies relevant contact events using non-collocated acoustic emissions (AE) sensing. By applying machine learning to benchtop-simulated training sets, we were able to identify the key features for identifying relevant contact events in RDS's full robotic system.
Links:
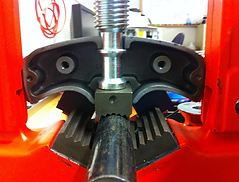
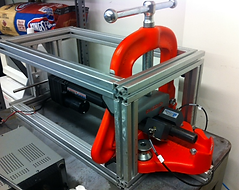
Photos above: our benchtop experimental setup replicating the RDS robot's features.
This video demonstrates how the robot and roughneck work together to join or break apart pipes during oil drilling operations.
RDS's autonomous oil drilling robot. We visited their manufacturing plant in Sandnes, Norway and got to see it in action!